- Sep 20, 2017
- 697
- 554
I found this alert a bit comical and I've heard of some people getting some wonky AI alerts, so I thought it would be cool to start a thread where we can share our AI capture fails!
Don't get me wrong, I love AI! I just recently learned how to install DeepStack and then Code Project's SenseAI, and before then I was paying for Sentry. I'm very thankful for being able to filter out the noise and only get alerted on things I find important. I'm no AI expert but it seems to work pretty darn well! But it can be a bit funny when something is misclassified.
I don't know about you, but I've never seen a person with wings before!
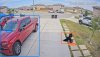
Stray cat! lol this one is a more understandable but still good nonetheless!
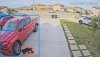
Another common one, but one that makes me chuckle a bit... When using the default model, I would get a lot of cars and trucks classified as boats! Would be even more funny if it was an old Cadillac or something like that.
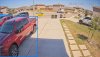
Anyway, hope to see your AI Capture Fails next! Keep it going!
Regards,
Philip
Don't get me wrong, I love AI! I just recently learned how to install DeepStack and then Code Project's SenseAI, and before then I was paying for Sentry. I'm very thankful for being able to filter out the noise and only get alerted on things I find important. I'm no AI expert but it seems to work pretty darn well! But it can be a bit funny when something is misclassified.
I don't know about you, but I've never seen a person with wings before!
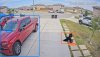
Stray cat! lol this one is a more understandable but still good nonetheless!
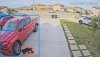
Another common one, but one that makes me chuckle a bit... When using the default model, I would get a lot of cars and trucks classified as boats! Would be even more funny if it was an old Cadillac or something like that.
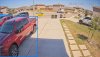
Anyway, hope to see your AI Capture Fails next! Keep it going!

Regards,
Philip